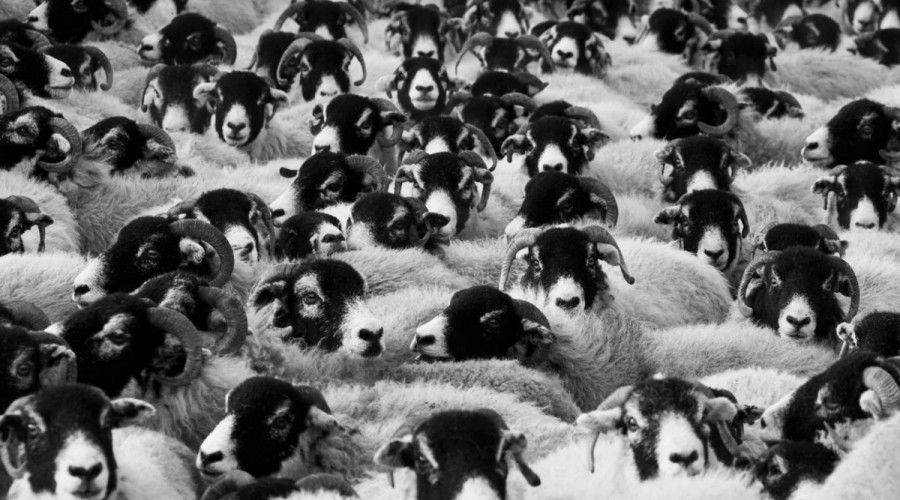
Academic Writing and ChatGPT
OpenAI’s Chat GPT has raised serious concerns in the academic community about students using AI-generated text to complete coursework. With the proliferation of increasingly smart AI models that are generative across all modalities, faculty are put in the unenviable position of having to determine, essentially, whether or not a student is earning their university degree on the strength of a succession of “deep fakes.”
Alongside the usual problems of plagiarism and its fellows, instructors fear confrontation with a reality in which evaluating the authenticity and merit of student products hinges on a correct guess at the “bot or not?” question. That’s a question that even AI has difficulty answering. With academic writing being at the heart of university course design and assessment, do professors even stand a chance in the face of powerful and adaptable AIs like ChatGPT? What’s to be done?
Probably nothing. With some adjustments to assessment practices and remaining confident in the face of fear, a lot of the “ChatGPT problem” simply goes away.
ChatGPT is less of a threat in the university context than is assumed, as long as faculty are serious about rigour in student research and communication. Ironically, there are two clear winners for survival against AI-generated text: the academic research paper and the emotionally-driven, first-person narrative. The white-hot danger zone lies in the middle between these two poles. For example, the simple expository essay is to be avoided at all costs at the university level. In addition, an emphasis on targeted assessment by faculty will help close the window on use and abuse of AI-generated text by students.
ChatGPT and the academic research paper: common ground
Although written assessments are the hallmark of the contemporary university experience, the purpose of a university is not to engage writing for the sake of writing: it is to contribute to knowledge creation and exploration. Knowledge creation and exploration require an emphasis on learning how to learn strategically, creatively, imaginatively, and critically, and then communicating learning for the purpose of sharing knowledge. Writing is just one tool among many for thinking and communicating in the academic community.
ChatGPT comes from the same DNA as academic writing. Literally. And they both run on the rules of natural language.
The thing about natural language being a system in its own right is that it works systematically. Anything that works systematically and predictably can, in theory, be replicated, given the right conditions and design. Languages have redundancy. The redundancy rate for English has been estimated at about 50%, which makes things like 2-D crossword puzzles and Wordle possible. AI programs like ChatGPT are possible for the same reason. The so-called Semantic Turn in AI has expanded the horizon of possibility, and developers have concentrated on leveraging the generalizable components of natural language as a system—combined with massive amounts of training data—to mimic very specific patterns of speech, thought, and behaviour. For this reason, within a short period of time, AI-generated text capabilities have moved from rudimentary spell-checkers to having convincing human-bot interactions on relationship platforms like Replika or with mental-health chatbots. The goal has been to match context with context, sentiment with sentiment, logic with logic, and so on.
Human verbal texts are instantiated in very specific ways, regardless of their modality. They become functional through sets of rules that accrue to them. The academic research paper in English, for example, is no different. It is formulaic, and rests on acceptable replication of established patterns of structure, argument, reasoning, and expression. There is almost no variation in tone or register, with some canonical adjustments for disciplinary focus. The system of transition or conjunction, in which logical relationships and meaningful connections between entities at clause, sentence, and paragraph level are made, consists of a selection of stock words and phrases that are deployed in a predictable manner. Lexical and semantic networks are leveraged in limited ways. Typically, a sense of originality in a research paper arises from the way in which it juxtaposes specific items from restricted fields of content across disciplines, in combination with interpretations that arise from the imposition of typical techniques of methodology and analysis.
On the whole, originality is hard to come by. But when a human writes, they are giving a particular kind of communicative performance driven by their own linguistic and intellectual agency in relation to human community. When ChatGPT does it, it is running code, and the linguistic and intellectual input of the humans involved is limited to data, design, and supervision.
Reality check
Like many bots of its kind, ChatGPT can potentially contribute to the destabilization of knowledge processes in and through the vagaries of its training data and design. Due to its dialogic format and appropriation of sentiment in order to mimic human interpersonal communication, it could have serious impact on personal mental and emotional states for users. Worse, in the long run, intensive and pervasive use of AIs like ChatGPT threaten to disrupt language development and related skills at the sociocultural, neurocognitive, and relational level for all humans.
Nevertheless, where it comes to student use of ChatGPT to achieve success on written assignments, university professors may have reason to downgrade their levels of concern—at least in the short run.
OpenAI’s latest version, GPT-4, remains limited in its scope for text-based outputs. Its touted benchmarks, like achieving the 99th percentile in the USA Biology Olympiad and the 90th percentile on the American Uniform Bar Exam (UBE), are not as terrifying as they may seem. The Biology Olympiad is a 50-minute, multiple-choice exam administered to high-schoolers, and completely reliant on established scientific knowledge; the written responses to the UBE questions require reprocessing of supplied text through rules of logic and law, expressed in highly repetitive and formulaic vocabulary. The examples of advanced natural language processing and problem-solving capabilities provided on the OpenAI site are classics of semantic logic. These domains are already a best-fit with AI at the design level.
ChatGPT requires a certain level of linguistic strategizing to drive the model towards a satisfying result: the more expert, specific, and linguistically agile the user, the better the output generated by the AI. Ultimately, the “chat” is command-and-response dressed up as a friendly conversation and, due to the current limitations of the bot, the efficiency-efficacy quotient in using ChatGPT for the generation of academically acceptable research papers or convincing first-person narratives is almost nil.
Students who attempt to write academic-standard research papers at the university level with ChatGPT will find that the same characteristics that produce negative results for human-generated papers are replicated in the bot’s outputs.
Concern versus performance
Let’s see how the concerns about ChatGPT match up with its actual performance.
Paraphrasing, summarizing, annotating: Since their advent, students have been using autoparaphrasers to transform their own writing and to disguise that of others. Autoparaphrasers are not terribly sophisticated, and student-generated paraphrase is questionable at the best of times. Arguably, ChatGPT is better than other free apps at producing a decent result. ChatGPT responds fairly well to text-dumping for the purposes of paraphrase or summary. It probably should do, because its autogenerated outputs amount to remixed plagiarism from somewhere. Like other autoparaphrasers, however, the result is telling: way too much of the original wording/sentence structure remains intact. ChatGPT could be used to summarize existing arguments or points-of-view in a field or on a topic, but the output would be largely devoid of appropriate references.
Autogenerated essays: ChatGPT can write a superficial, syntactically and semantically sound short “essay” on any given topic. However, ChatGPT does not provide references of any kind unless prompted. When it does provide references, they are often inaccurate, wrong, or so vague as to be unhelpful, barring the user driving it on to better results. Since the app does not have access to academic research databases, and relies on its training data, there is a hard limit to the resources it can draw on to build in any more specificity. This situation could change in the future. There may then be some cause to worry. For these reasons, simple expository, descriptive, definitional essays, et cetera, should be avoided in favour of the academic research essay.
Plagiarism: A hallmark of ChatGPT outputs is an obvious lack of references, alongside virtually no indication or use of direct quotations. By the same token, a student paper lacking in these features is likely the result of unoriginal writing. Students using ChatGPT to generate papers with a research component requiring a strict documentation practice would have to retroactively fill this in through falsification and/or misappropriation of sources and references, a strategy that is a traditional signal of inauthentic or lifted content.
AI-generated text requires AI detection: Another AI platform will not be effective, in the long run, at deciding “bot or not.” Even if it does suggest that a student used an AI language model like ChatGPT or similar to generate text, there is no ethical way of establishing this use definitively.
Written assessments are now obsolete: Creative writing is perhaps less threatened by ChatGPT than academic writing, however, that situation is likely to change. If assessments are focused on the demonstration of originality in thinking and facility with finding, applying, and analyzing research sources to support that thinking, then it is less likely that a student will be able to use ChatGPT successfully to complete assignments. Assessments need to be targeted to specific higher-level skills, and not primarily focused on “good grammar” and “logical flow,” which represent the syntactic and semantic principles upon which text generators are designed.
Course content will become irrelevant: Written assignments are not always the best measure of student absorption of course content. If this is the assessment goal, opt for multiple-choice tests or exams that evaluate for that outcome. Create questions that require students to problem-solve their way to an answer. Bringing oral examinations back into the university environment may also be an important strategy going forward.
The literary classics are a no-go: AI can do the present just as well as the past, within the confines of its training data, so it’s not true that sticking to more recent and/or obscure literature will mitigate the use of AI for literary analysis, especially in the long run.
“Bot or not?” spotting
ChatGPT has some readily identifiable quirks. These include:
- bet-hedging: when it doesn’t have enough data or a clear decision-path, the bot responds to even the most obvious queries with equivocating, non-committal phrases, e.g. “Shakespeare did not write extensively about quantum physics”
- circular conclusions: the bot fabricates “closed loop,” highly repetitive conclusions, and does so within a short space of textual output
- an inability to generate academic references: ChatGPT does not supply academic references or citations in support of its claims or information; it will make vague and limited references to authors and works for general descriptive purposes
- a lack of direct quotation: the bot does not generate (or acknowledge) direct quotations
- narrativizing rather than analyzing data (facts, events, concepts, information): ChatGPT rates low on critical thinking versus listing, describing, recounting, and matching content, mirroring cues in the user’s chat
- providing misleading and/or superficial analysis: the bot does not provide in-depth analysis to any reasonable academic standard
- inaccurate facts: the bot is limited in its presentation of facts by its training data set and interaction with the user
- weirdly contradictory claims, examples, and evidence: the bot sometimes hits a wall in the face of options in the data and/or lack of control in supervision or programming
- vague wording: ChatGPT, somewhat like the student whose essay response simply restates the exam question in five different ways, imitates and bet-hedges in its outputs; coupled with its penchant for superficial analysis, there are sentences that, while devoid of fatal syntactical or semantic errors, are lacking in relevance and depth of meaning
- repetitive constructions: besides generally being very repetitious, ChatGPT is over-reliant on front-ended, one-word conjunctions like “However” and “Overall”
- paratactic style: the bot tends to avoid hypotaxis, sticking to simple or compound sentences in its output
- argumentation by demarcation of logical boundaries in discrete, extremely short paragraphs: there is relatively little sophistication in the development of paragraphs, which are often 2 or 3 sentences long. The typical ChatGPT pattern is: para 1, opening proposition; para 2, contrastive or comparative claim (e.g. “However,” “Likewise,”); para 3, illustration (e.g. “For example,”); para 4, circular conclusion (e.g. “Overall,” with repetition of significant elements of 1, 2, and 3).
Even if the “bot or not?” question can be guessed with relative security based on these diagnostics, it is likely not provable, at least not within the bounds of ethics. The good news, however, is that the characteristics that make ChatGPT’s “essays” very bad also make papers written by humans very bad—and equally deserving of very bad grades. Therefore, professors should feel confident in continuing to dole out abysmal marks for all of the usual reasons and more, whether “bot or not.”
What now?
ChatGPT is scary and chilling on many levels, not least for the disastrous levels of data-mining its use entails. At the same time, it is fun and exciting, and could be used in some instructive ways to help students in higher learning develop or deepen various skills. Here are some random examples of activities for which ChatGPT could be useful:
- create, refine, and explore research topics and questions
- compare, contrast, and evaluate the bot’s output using various modes and levels of analysis
- identify and evaluate “common knowledge” versus “specialized knowledge” across disciplines
- trace sources and resources that may have contributed to the bot’s text
- identify how to rehabilitate or transform the bot’s output into a genuine academic research paper
- perform textual analysis (syntactic, semantic, etc.) on outputs
- examine syntactic and semantic differences between regenerated responses, paraphrases, etc. using a variety of source texts, including the bot’s own “original” outputs
Of course, playing around with a fairly powerful AI does come with significant caveats. Use responsibly!
Invitation to the ChatGPT Party
If you’re looking for the experimental evidence behind any of the assertions above, check out ChatGPT Party episodes 1-6, in which I challenge the bot to a series of tasks with interesting results, including a limerick for St. Patrick’s Day!
Citation:
Reid, Jennifer. “Academic Writing and ChatGPT.” Winnsox, vol. 4 (2023).
ISSN 2563-2221